The tragic crashes of two Boeing 737 Max 8, one in Indonesia on October 28, 2018 and the other in Ethiopia on March 10, 2019, are highlighting the risks and uncertainties introduced by software in automated systems. The investigations are proceeding despite Ethiopia’s decision not to cooperate with the United States National Transportation Safety Board (NTSB). Reports of US pilots encountering control problems with the Max 8’s Maneuvering Characteristics Augmentation System (MCAS) came to light after the latest accident, although none of these incidents led to an in-flight emergency. One pilot also expressed frustration with the flight manual and poor communications from the manufacturer. “I am left to wonder: what else don’t I know?... The Flight Manual is inadequate and almost criminally insufficient.[1]”
It’s likely that more experience and better training helped US crews recognize MCAS problems and maintain control of their planes. Boeing was listening and had already scheduled an upgrade to the cockpit software before the latest crash. The accidents led the national leadership of the Airline Pilot Association Union (ALPA) and branches at American and Southwest to complain that Boeing had not disclosed enough information about the MCAS to their pilots. They saw it as a potential safety issue.
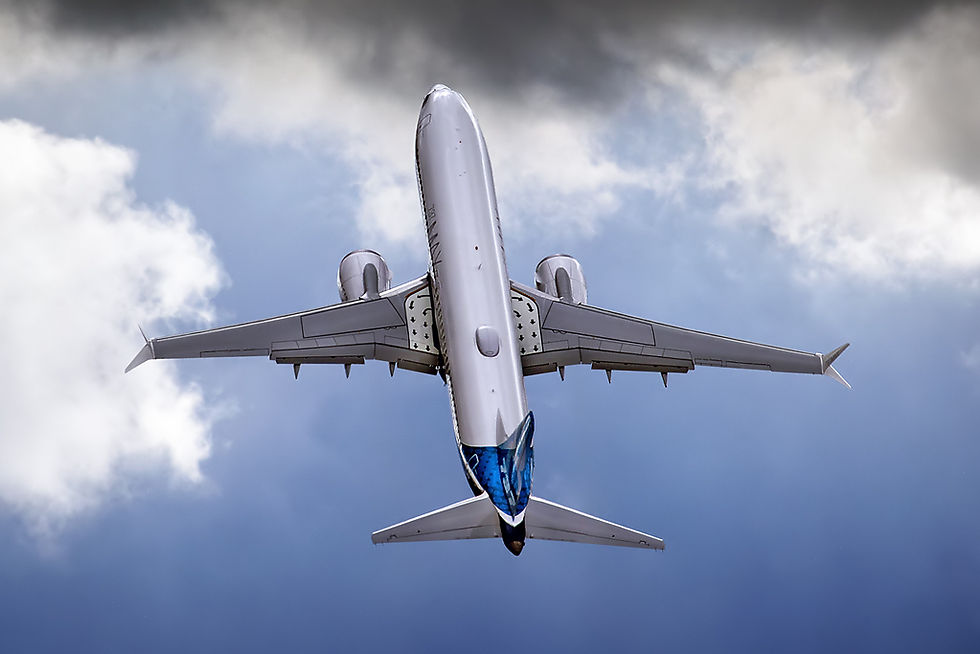
Not all pilots agreed with ALPA and its American and Southwest branches. Captain Todd Insler, head of the ALPA branch at United Airlines, broke rank with the national union and colleagues at American and Southwest. His view is that pilots need the knowledge, training, and ability to fly their aircraft and take control when something goes wrong. He points out that, unlike Airbus, Boeing exposes more of its automated systems to pilot control. This approach allows pilots to circumvent automation in response to unexpected system problems and behaviors. In his view, “The story [with the Ethiopian crash] is not why we didn’t know about (the new system), it’s why the pilots didn’t fly the plane.[2]”
Problems with software, pilot training, and automated flight control systems are not new or unique to the Max 8. There have been many incidents where pilots were not aware of or did not fully understand programmed behaviors in their flight control computers. Some of these incidents ended tragically. An early example was the crash of China Airlines Flight 140 on April 26, 1994, at Nagoya Airport in Japan in which 264 died and 7 were seriously injured. The incident began when the Pilot Flying (PF) inadvertently activated the GO-AROUND mode on the Airbus A300-600R. A Go-Around is an action used by pilots to abort a landing by climbing to altitude and then returning for another landing attempt.
The A300 had the most automated flight control system in the industry at the time and could execute Go-Arounds with minimal pilot action. The system had been engineered to prevent human errors by overriding pilot inputs that could place aircrafts in potentially unsafe configurations. The crew of Flight 140 attempted a landing while the A300 was in GO-AROUND MODE. Their control inputs conflicted with the flight control computers’ programming. Specifically, the pilots were trying to lower the plane’s nose as they approached the runway, but the A300 flight controls overrode their input and raised plane’s nose as part of executing a Go-Around. A physical struggle ensued in which the pilots tried to force the plane to respond to their input, while the flight control software was forcing the controls to execute the unintentionally selected Go-Around directive. It ended in catastrophe when the conflict caused the plane to stall and crash[3],[4].
Fourteen years later, on March 1, 2008, Lufthansa Flight LH 044 nearly ended in tragedy as the Airbus A320 was attempting to land in heavy cross winds at Hamburg’s airport. The crew suddenly found that their plane’s flight controls were not responsive to pilot input. They executed a successful manual Go-Around maneuver and safely landed minutes later. Their incident report and video of the near crash lead to an official investigation by Germany’s Federal Bureau of Aircraft Accident Investigation (BFU).
The BFU determined that an intentional design feature in the A320’s flight control system software was responsible for the plane’s unexpected behavior. The initial landing attempt had caused the plane to bounce on the runway, which depressed the landing gear. This action switched the flight control computers’ operating mode from Flight to Ground. The software responded by reducing power to the ailerons and limiting pilot control. The Lufthansa crew did an outstanding job recovering from the situation by increasing power and aborting the landing.
The feature that nearly caused the crash was designed to enhance passenger experience by smoothing the plane’s ground behavior after landing. It was never intended to be active in flight. The BFU concluded that the pilots were not aware and had not been trained on this feature. Airbus later issued a pilot advisory, and airlines updated their operations and training procedures to ensure their pilots were aware of and practiced on this peculiar characteristic of the A320.
A more disturbing incident the same year involved Qantas Airlines Flight 72 from Singapore to Perth, Australia on October 7, 2008. The Airbus A330-300 carrying 303 passengers and 12 crew was under the command of an American Navy Veteran, Captain Kevin Sullivan, and two Australians, First Officer Peter Lipsett and Second Officer Ross Hales. They were cruising at 37,000 feet on a clear day over the Indian Ocean, when the autopilot suddenly disengaged, forcing Sullivan to take manual control. Seconds later the plane’s Electronic Centralized Aircraft Monitor (ECAM) began issuing overspeed and stall warnings, two conditions that should never occur at the same time. The crew consulted their instruments and visually checked their plane’s attitude to verify that it was not in a stall or overspeed condition.
A few minutes later (12:42:27 PM Western Australia Time), the plane’s nose suddenly pitched down 8.4 degrees triggering an abrupt 150-foot drop in just a few seconds. The sudden loss of altitude sent unfastened people and objects crashing onto overhead bins and cabin ceiling. The A330’s flight controls were initially unresponsive, but Sullivan and his crew managed to regain control. The plane dropped a total of 690 feet in 23 seconds, so the crew began a slow, controlled climb to their assigned 37,000-foot cruising altitude.
Shortly after recovering from the dive (12:45:08 PM WAT), the A330’s nose again pitched down 3.5 degrees and began a second dive. This time dropping 400 feet in 15 seconds before the pilots regained control and returned to cruising altitude. They then declared an in-flight emergency after learning of multiple passenger and cabin crew injuries and were cleared to divert to a closer airport at Learmonth, where they landed at 13:32 WAT. The incident caused a total of 315 injuries, 12 of them serious[5]. The investigation by Australia’s Transport Safety Bureau did not find the specific cause of the flight upset. It did uncover similar incidents involving other A330s. Airbus later issued an update to their ECAM software to prevent recurrence of these problems.
Less than a year after the Lufthansa and Qantas incidents, on June 1, 2009, Air France Flight 447 plunged into the Atlantic during a flight from Rio de Janeiro, Brazil, to Paris. The Airbus A330-200 was flying through severe weather when the pitot tubes that measure airspeed were affected by ice, triggering conflicting readings. The plane’s Flight Management System software could not resolve the airspeed discrepancies and the autopilot disconnected. Flying at night and without a visible horizon, the crew lost situational awareness, put the plane in a stall condition, and failed to recover in time. Flight 447 crashed into the ocean after a three-minute plunge from 38,000 feet, killing all aboard. The data from the Flight and Voice Recorders suggested that the pilots were trying to fly their computers, instead of their aircraft[6].
Pilots are not the only equipment operators who are sometimes bedeviled by the complexities of software. Modern cars have dozens of microprocessors executing between one and two hundred million lines of code[7]. Bugs and confusing undocumented features have triggered massive recalls by nearly all manufacturers including GM, Ford, Chrysler, Mercedes-Benz, BMW, Volkswagen, Hyundai, Honda, and Toyota. Software defects in vehicles' electronic control units (ECUs) have been on the rise for years and now account for about 20% of all recalls.
The Uber self-driving car accident that killed a pedestrian in Tempe, Arizona on March 18, 2018, illuminated growing conflicts between automation and the human ability to intervene in emergencies. Our own investigation determined that assumptions about the ability of a backup driver to intervene in emergencies were fundamentally flawed. We concluded that the Uber driver could not have achieved situational awareness, taken control, and intervened in time to prevent the accident. The Uber operational model internalized safety problems through unrealistic assumptions about automation and human performance[8],[9].
Software-related safety issues are not restricted to large, automated planes, vehicles, and industrial equipment. Medical devices regulated by the US Food and Drug Administration (FDA) have also been broadly affected by software defects. A study of medical device recalls between 2011 and 2015 found that a “total of 627 software devices (1.4 million units) were subject to recalls, with 12 of these devices (190,596 units) subject to the highest‐risk recalls.[10]” These types of software-related problems can be difficult to find because their effects on patients and diagnostics are not easily detectable.
Summary and implications
It’s against this backdrop that artificial intelligence (AI) technologies are growing in importance as they spread across applications and industries in the global economy. AI will deliver more capable levels of system automation and autonomous decision-making than previous generations of software. The benefits are compelling, but not without risks and uncertainties.
Software and software bugs have unique characteristics that are distinctly different from defects in physical system components. Software behaviors can change, and new bugs introduced every time new code is installed. I’ve worked in environments where undetected defects were distributed within a few hours to millions of devices during a software update. The bugs only became evident after users jammed customer service lines to report problems and seek solutions.
Unplanned behaviors and undocumented features can hide in software for long periods until they are triggered by uncommon sets of factors and conditions. This is where operator training and experience can prevent catastrophes. Unfortunately, as highlighted in the China Airlines Fight 140, Lufthansa LH 044, and Qantas 72 incidents, some automated systems make it difficult for operators to quickly reestablish manual control. The value of training and experience is diminished when they can’t be quickly and effectively translated into action.
Implications
Software does not exercise judgment outside of its programmed and programming constraints. They coldly follow their programming and logic as designed, even when it leads to undesirable outcomes. Modern systems get it right most of the time, but no amount of testing will deliver certainty. After all, these systems are designed and programmed by fallible human beings, who introduce errors and unplanned behaviors, and sometimes fail to detect them. It’s something AI pioneer and Nobel Laureate Herbert Simon pointed out many years ago when he asserted that “no number of viewings of white swans can guarantee that a black one will not be seen next[11].”
Safety organizations and government agencies like the US National Transportation Safety Board (NTSB) will face increasing challenges in deciphering software bugs and capturing undocumented behaviors in future accident investigations. Software on complex equipment like aircraft and cars is usually developed by multiple companies that operate under their own structures and standards. Producing a final product or system involves integrating subsystems and components, each with its own microprocessors and code. Testing is critical, but experience and growing system complexity suggest that unwanted bugs and behaviors will always be present.
Artificial intelligence will add another level of complexity that will challenge manufacturers, system integrators, operators, and regulators. That’s because AI technologies like machine learning create behaviors that are non-deterministic. In practice, this means that AI software learns during operations and can change their behaviors to improve performance. Decoding behaviors in these systems can be daunting, although in theory their ability to adapt and change will be limited by designers and engineers. It’s even more challenging in complex systems composed of subsystems running their own AI algorithms because their interactions can create unplanned adaptive systems-of-systems environments. These environments are notoriously difficult to control. Engineers and mathematicians usually describe their behaviors as locally controllable and globally influenced by operator input.
Finally, there are growing concerns over how human operators interact with automated/autonomous equipment like modern aircraft and self-driving cars. Humans are the ultimate complex adaptive system in that there are no guarantees for how we will behave (and not behave) in response to events. In the early 1900s, operator mistakes and accidents were blamed on people, who were often labeled as ‘accident prone’ and removed from hazardous environments. The growing complexity of equipment and operator-induced accidents during the Second World War caused a reassessment and reconsideration of the root causes of accidents. Engineers, accident investigators, and operators eventually recognized that poor human-machine interfaces were the primary or root causes of many accidents. These insights lead to a new discipline, human factors engineering (HFE)[12].
HFE focuses on how systems work in practice, with fallible human beings at the controls. The objectives of the discipline are to improve ease of use and reduce the probability of mistakes, particularly those that undermine safety. HF engineers analyze how well system designs account for human strengths and limitations. Well-designed systems make it easier for humans to operate systems and equipment, are tolerant of human error, and help operators recognize and recover when the unexpected happens.
Autonomous systems, advanced automation, and artificial intelligence are challenging human factor design principles because they shift the locus of decision-making and control from operators to software. This shift has created a gap in HFE practices because we don’t have conclusive evidence on which human-machine interface design principles work best in systems that place humans in caretaker mode. The rapid pace of innovation further complicates the process by continuously changing the balance of control between human operators and software. The experience base is sufficiently developed to cast concerns over autonomous/automated system interfaces, operations, and training. Unfortunately, it remains a work in progress in terms of providing new solutions for emerging operational environments[13].
The NTSB will likely need some form of National Transportation Software Board to help them cope with increasingly complex software in highly automated and autonomous systems. Their challenges will be aggravated by dozens of software versions installed across global fleets and platforms. Growing experience operating systems with embedded software suggests that we are a long way from understanding, coping with, and managing the risks and uncertainties they introduce.
Image reference
Image courtesy of Wikipedia and Oleg V. Belyakov under the Creative Commons Attribution-Share Alike 3.0 Unported license.
References
Image courtesy of Wikipedia Commons - Acefitt
[1] David Koenig, Michael Sisak, Pilots have reported issues in US with new Boeing jet, March 12, 2019, https://apnews.com/0cd5389261f34b01a7cbdb1a12421e27
[2] Dominic Gates, Dispute arises among US pilots on Boeing 737 Max system linked to crash, November 16/17 2018, The Seattle Times, https://www.seattletimes.com/business/boeing-aerospace/dispute-arises-among-u-s-pilots-on-boeing-737-max-system-linked-to-lion-air-crash/
[3] Japan Accident Investigation Commission, Aircraft Accident Investigation Report 96-5, July 19, 1996, http://sunnyday.mit.edu/accidents/nag-3.html
[4] Nakao Masayuki, Case details, China Airlines Flight 140, Association for the Study of Failure, http://www.shippai.org/fkd/en/cfen/CA1000621.html
[5] Aviation Occurrence Investigation, AO-2008-070, Final, Australian Transport Safety Bureau, 2011, https://www.atsb.gov.au/media/3532398/ao2008070.pdf
[6] Dean Macris, Ozzie Paez, Automation and the unaware caretakers, May 1, 2018, Ozzie Paez Research, https://www.ozziepaezresearch.com/single-post/2018/04/30/Automation-and-unaware-caretakers
[7] Phil Koopman, Potentially deadly automotive software defects, September 25, 2018, Better embedded system software, https://betterembsw.blogspot.com/2018/09/potentially-deadly-automotive-software.html
[8] Ozzie Paez, Dean Macris, Who’s responsible for Uber’s self-driving vehicle accident, June 15, 2018, Ozzie Paez Research, https://www.ozziepaezresearch.com/single-post/2018/06/15/UberSelfDrivingVehicleAccident
[9] Ozzie Paez, Dean Macris, The fatal Uber self-driving car crash – update, July 12, 2018, Ozzie Paez Research, https://www.ozziepaezresearch.com/single-post/2018/07/12/The-fatal-Uber-self-driving-car-crash---update
[10] Ronquillo JG, Zuckerman DM. Software-Related Recalls of Health Information Technology and Other Medical Devices: Implications for FDA Regulation of Digital Health. Milbank Q. 2017;95(3):535-553., https://www.ncbi.nlm.nih.gov/pmc/articles/PMC5594275/
[11] Hebert A Simon, Reason in Human Affairs, The Harry Camp Lectures at Stanford University, 1982, Kindle Edition, Location 753, Stanford University Press, location 49.
[12] Sidney Dekker, Safety differently – human factors for a new era, 2nd Edition, pgs. 1 – 5 , Kindle Edition, 2015, CRC Press.
[13] Ozzie Paez, The increasingly confusing language of automation, May 17, 2018, Ozzie Paez Research, https://www.ozziepaezresearch.com/single-post/2018/05/17/The-growing-language-of-automation